Assistant Professor of Engineering Maggie Delano on the Trouble with Sex and Machine Learning
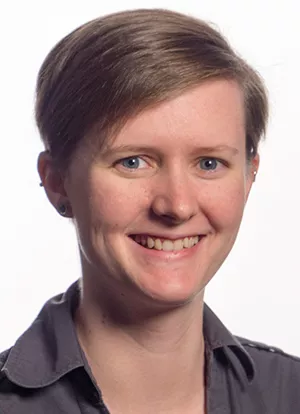
Machine learning holds the promise of helping researchers create more inclusive, dynamic systems and helping clinicians provide better care, improving patient experience and outcomes. But the data being used to create these systems comes from current electronic health records (EHRs), and as Assistant Professor of Engineering Maggie Delano has found in their new research, this means trouble — sex trouble.
With "Sex Trouble: Sex/Gender Slippage, Sex Confusion, and Sex Obsession in Machine Learning Using Electronic Health Records," published in the journal Patterns, Delano and their colleague Kendra Albert have found that rather than correcting existing problems, data from EHRs could just be perpetuating these problems.
“[Gender and sex] are appealing to use for studies, as the assumption is that they are a ‘clean’ field to use. However, we argue that sex/gender needs to be considered in context, more like a family history field might be,” says Delano. In the case of a patient with diabetes, for example, increasing data richness would not only indicate that the patient has diabetes but include info on the type of diabetes, date of diagnosis, and recent blood test results.
Through this research, Delano and Albert have identified three concepts that best capture the most common problems around sex and gender in research: sex/gender slippage, sex confusion, and sex obsession.
Sex/gender slippage is conflating sex terms and gender terms. For example, when someone’s gender is identified as male or female instead of man or woman. Male and female are terms to define sex, not gender.
Sex confusion is the idea that any sex variable, such as sex assigned at birth or current sex, holds several different meanings and interpretations and do not correspond to particular body parts or active hormones.
And finally, sex obsession is the idea that the most helpful variable for defining someone’s gender or sex is sex assigned at birth. Or, as Delano explains, “Sex obsession is the idea that even as certain parts of society engage with the complexities of sex/gender, medical systems ‘double down’ on more simplistic representations.”
Delano says the most common problem is sex/gender slippage, but that is because sex confusion and sex obsession are harder to identify without clarity from researchers.
“If a researcher does not say how they are using sex in a study, unless they make common mistakes such as conflating sex with gender, we can’t know for sure that sex confusion is occurring,” explains Delano. "This is also true for sex obsession: the idea of the importance of sex assigned at birth [being greater than] other variables was only explicitly stated in a few papers specifically related to EHRs."
Delano draws on the work of Afsaneh Rigot and advocates for designing models and systems for people on the margins of gender and sex rather than the center.
“By centering trans and non-binary people, rather than seeing them as an edge case to be ignored, better health care can be made available to everyone. Relevant information will depend on the context, but information such as whether a patient is currently or has in the past taken hormones, has had certain surgeries, etcetera, will be helpful,” says Delano. “One implementation of this is what is called an organ inventory, which allows clinicians to view the presence or absence of organs from a patient. This is helpful to quickly determine, for example, if a patient currently has a uterus or testicles.”
Important strides have been made in trying to facilitate a more complex understanding of gender and sex in current medical data collection practices, including the creation of the NIH’s Office of Sexual & Gender Minority Research, the requirement for EHRs used in certain contexts to collect information on sexual orientation and gender identity (SOGI), and last year’s Health and Human Services announcement that SOGI data would now be included in the United States Data Core, which sets the standards for medical record systems. Delano’s research suggests while helpful, these steps are not enough, and that machine learning researchers need to stop relying on sex assigned at birth completely and start accounting for richer gender experiences.
She hopes this research will inspire clinicians and other researchers to keep context and patient goals in mind to develop the best systems or provide the best care.
“What assumptions are you making? What variables are ‘standing in’ for other variables? Are those variables actually measuring what you think they are measuring? If you don’t know, what steps do you need to take to find out? How can you expand your network to better educate yourself?” asks Delano. “All change needs to start from answering these questions. As each field develops better understanding, improvements and recommendations can be made, and all patients will benefit.”